- Main
- Biology and other natural sciences
- Essential bioinformatics
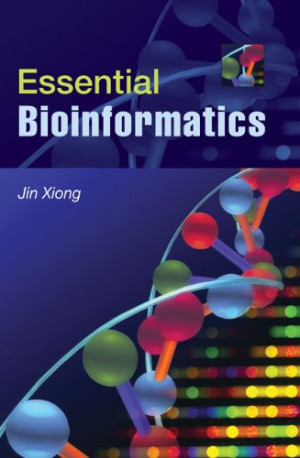
Essential bioinformatics
Xiong J.你有多喜欢这本书?
下载文件的质量如何?
下载该书,以评价其质量
下载文件的质量如何?
Essential Bioinformatics is a concise yet comprehensive textbook of bioinformatics, which provides a broad introduction to the entire field. Written specifically for a life science audience, the basics of bioinformatics are explained, followed by discussions of the state-of-the-art computational tools available to solve biological research problems. All key areas of bioinformatics are covered including biological databases, sequence alignment, genes and promoter prediction, molecular phylogenetics, structural bioinformatics, genomics and proteomics. The book emphasizes how computational methods work and compares the strengths and weaknesses of different methods. This balanced yet easily accessible text will be invaluable to students who do not have sophisticated computational backgrounds. Technical details of computational algorithms are explained with a minimum use of mathematical formulae; graphical illustrations are used in their place to aid understanding. The effective synthesis of existing literature as well as in-depth and up-to-date coverage of all key topics in bioinformatics make this an ideal textbook for all bioinformatics courses taken by life science students and for researchers wishing to develop their knowledge of bioinformatics to facilitate their own research.
内容类型:
书籍年:
2006
出版社:
CUP
语言:
english
页:
362
ISBN 10:
0521840988
ISBN 13:
9780521840989
文件:
PDF, 5.65 MB
您的标签:
IPFS:
CID , CID Blake2b
english, 2006
添加到我的图书馆
- Favorites
在1-5分钟内,文件将被发送到您的电子邮件。
该文件将通过电报信使发送给您。 您最多可能需要 1-5 分钟才能收到它。
注意:确保您已将您的帐户链接到 Z-Library Telegram 机器人。
该文件将发送到您的 Kindle 帐户。 您最多可能需要 1-5 分钟才能收到它。
请注意:您需要验证要发送到Kindle的每本书。检查您的邮箱中是否有来自亚马逊Kindle的验证电子邮件。
正在转换
转换为 失败
关键词
关联书单
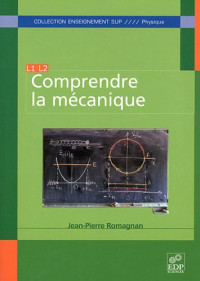
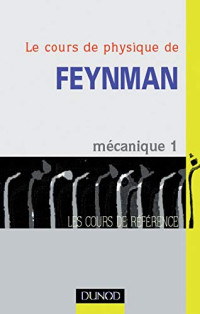
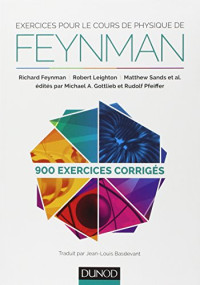
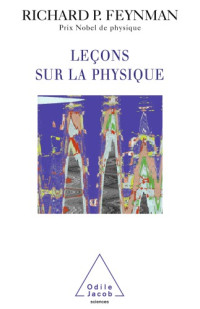
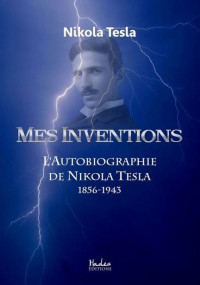
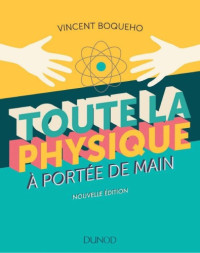
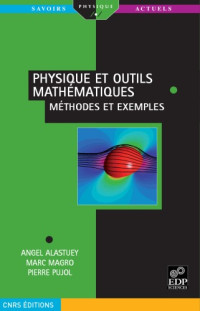
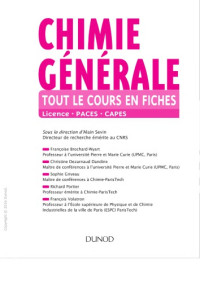
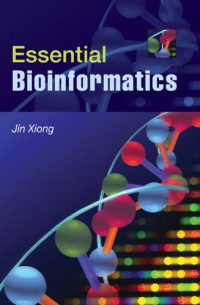
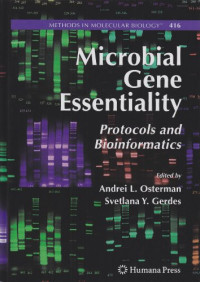
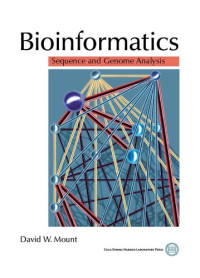
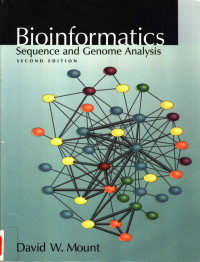
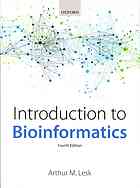
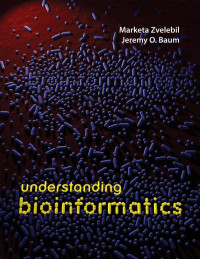
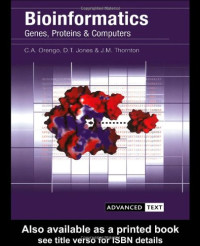
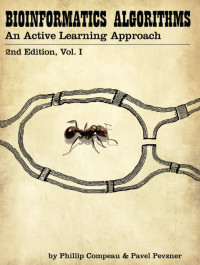
P1: JZP 0521840988pre CB1022/Xiong 0 521 84098 8 January 10, 2006 This page intentionally left blank ii 15:7 P1: JZP 0521840988pre CB1022/Xiong 0 521 84098 8 January 10, 2006 ESSENTIAL BIOINFORMATICS Essential Bioinformatics is a concise yet comprehensive textbook of bioinformatics that provides a broad introduction to the entire field. Written specifically for a life science audience, the basics of bioinformatics are explained, followed by discussions of the stateof-the-art computational tools available to solve biological research problems. All key areas of bioinformatics are covered including biological databases, sequence alignment, gene and promoter prediction, molecular phylogenetics, structural bioinformatics, genomics, and proteomics. The book emphasizes how computational methods work and compares the strengths and weaknesses of different methods. This balanced yet easily accessible text will be invaluable to students who do not have sophisticated computational backgrounds. Technical details of computational algorithms are explained with a minimum use of mathematical formulas; graphical illustrations are used in their place to aid understanding. The effective synthesis of existing literature as well as in-depth and up-to-date coverage of all key topics in bioinformatics make this an ideal textbook for all bioinformatics courses taken by life science students and for researchers wishing to develop their knowledge of bioinformatics to facilitate their own research. Jin Xiong is an assistant professor of biology at Texas A&M University, where he has taught bioinformatics to graduate and undergraduate students for several years. His main research interest is in the experimental and bioinformatics analysis of photosystems. i 15:7 P1: JZP 0521840988pre CB1022/Xiong 0 521 84098 8 January 10, 2006 ii 15:7 P1: JZP 0521840988pre CB1022/Xiong 0 521 84098 8 January 10, 2006 Essential Bioinformatics JIN XIONG Texas A&M University iii 15:7 cambridge university press Cambridge, New York,; Melbourne, Madrid, Cape Town, Singapore, São Paulo Cambridge University Press The Edinburgh Building, Cambridge cb2 2ru, UK Published in the United States of America by Cambridge University Press, New York Information on this title: © Jin Xiong 2006 This publication is in copyright. Subject to statutory exception and to the provision of relevant collective licensing agreements, no reproduction of any part may take place without the written permission of Cambridge University Press. First published in print format 2006 isbn-13 isbn-10 978-0-511-16815-4 eBook (EBL) 0-511-16815-2 eBook (EBL) isbn-13 isbn-10 978-0-521-84098-9 hardback 0-521-84098-8 hardback isbn-13 isbn-10 978-0-521-60082-8 0-521-60082-0 Cambridge University Press has no responsibility for the persistence or accuracy of urls for external or third-party internet websites referred to in this publication, and does not guarantee that any content on such websites is, or will remain, accurate or appropriate. P1: JZP 0521840988pre CB1022/Xiong 0 521 84098 8 January 10, 2006 Contents Preface ■ ix SECTION I 1 INTRODUCTION AND BIOLOGICAL DATABASES Introduction ■ 3 What Is Bioinformatics? ■ 4 Goal ■ 5 Scope ■ 5 Applications ■ 6 Limitations ■ 7 New Themes ■ 8 Further Reading ■ 8 2 Introduction to Biological Databases ■ 10 What Is a Database? ■ 10 Types of Databases ■ 10 Biological Databases ■ 13 Pitfalls of Biological Databases ■ 17 Information Retrieval from Biological Databases ■ 18 Summary ■ 27 Further Reading ■ 27 SECTION II 3 SEQUENCE ALIGNMENT Pairwise Sequence Alignment ■ 31 Evolutionary Basis ■ 31 Sequence Homology versus Sequence Similarity ■ 32 Sequence Similarity versus Sequence Identity ■ 33 Methods ■ 34 Scoring Matrices ■ 41 Statistical Significance of Sequence Alignment ■ 47 Summary ■ 48 Further Reading ■ 49 4 Database Similarity Searching ■ 51 Unique Requirements of Database Searching ■ 51 Heuristic Database Searching ■ 52 Basic Local Alignment Search Tool (BLAST) ■ 52 FASTA ■ 57 Comparison of FASTA and BLAST ■ 60 Database Searching with the Smith–Waterman Method ■ 61 v 15:7 P1: JZP 0521840988pre vi CB1022/Xiong 0 521 84098 8 CONTENTS Summary ■ 61 Further Reading ■ 62 5 Multiple Sequence Alignment ■ 63 Scoring Function ■ 63 Exhaustive Algorithms ■ 64 Heuristic Algorithms ■ 65 Practical Issues ■ 71 Summary ■ 73 Further Reading ■ 74 6 Profiles and Hidden Markov Models ■ 75 Position-Specific Scoring Matrices ■ 75 Profiles ■ 77 Markov Model and Hidden Markov Model ■ 79 Summary ■ 84 Further Reading ■ 84 7 Protein Motifs and Domain Prediction ■ 85 Identification of Motifs and Domains in Multiple Sequence Alignment ■ 86 Motif and Domain Databases Using Regular Expressions ■ 86 Motif and Domain Databases Using Statistical Models ■ 87 Protein Family Databases ■ 90 Motif Discovery in Unaligned Sequences ■ 91 Sequence Logos ■ 92 Summary ■ 93 Further Reading ■ 94 SECTION III 8 GENE AND PROMOTER PREDICTION Gene Prediction ■ 97 Categories of Gene Prediction Programs ■ 97 Gene Prediction in Prokaryotes ■ 98 Gene Prediction in Eukaryotes ■ 103 Summary ■ 111 Further Reading ■ 111 9 Promoter and Regulatory Element Prediction ■ 113 Promoter and Regulatory Elements in Prokaryotes ■ 113 Promoter and Regulatory Elements in Eukaryotes ■ 114 Prediction Algorithms ■ 115 Summary ■ 123 Further Reading ■ 124 SECTION IV 10 MOLECULAR PHYLOGENETICS Phylogenetics Basics ■ 127 Molecular Evolution and Molecular Phylogenetics ■ 127 Terminology ■ 128 Gene Phylogeny versus Species Phylogeny ■ 130 January 10, 2006 15:7 P1: JZP 0521840988pre CB1022/Xiong 0 521 84098 8 vii CONTENTS Forms of Tree Representation ■ 131 Why Finding a True Tree Is Difficult ■ 132 Procedure ■ 133 Summary ■ 140 Further Reading ■ 141 11 Phylogenetic Tree Construction Methods and Programs ■ 142 Distance-Based Methods ■ 142 Character-Based Methods ■ 150 Phylogenetic Tree Evaluation ■ 163 Phylogenetic Programs ■ 167 Summary ■ 168 Further Reading ■ 169 SECTION V 12 STRUCTURAL BIOINFORMATICS Protein Structure Basics ■ 173 Amino Acids ■ 173 Peptide Formation ■ 174 Dihedral Angles ■ 175 Hierarchy ■ 176 Secondary Structures ■ 178 Tertiary Structures ■ 180 Determination of Protein Three-Dimensional Structure ■ 181 Protein Structure Database ■ 182 Summary ■ 185 Further Reading ■ 186 13 Protein Structure Visualization, Comparison, and Classification ■ 187 Protein Structural Visualization ■ 187 Protein Structure Comparison ■ 190 Protein Structure Classification ■ 195 Summary ■ 199 Further Reading ■ 199 14 Protein Secondary Structure Prediction ■ 200 Secondary Structure Prediction for Globular Proteins ■ 201 Secondary Structure Prediction for Transmembrane Proteins ■ 208 Coiled Coil Prediction ■ 211 Summary ■ 212 Further Reading ■ 213 15 January 10, 2006 Protein Tertiary Structure Prediction ■ 214 Methods ■ 215 Homology Modeling ■ 215 Threading and Fold Recognition ■ 223 Ab Initio Protein Structural Prediction ■ 227 CASP ■ 228 Summary ■ 229 Further Reading ■ 230 15:7 P1: JZP 0521840988pre viii CB1022/Xiong 0 521 84098 8 CONTENTS 16 RNA Structure Prediction ■ 231 Introduction ■ 231 Types of RNA Structures ■ 233 RNA Secondary Structure Prediction Methods ■ 234 Ab Initio Approach ■ 234 Comparative Approach ■ 237 Performance Evaluation ■ 239 Summary ■ 239 Further Reading ■ 240 SECTION VI 17 GENOMICS AND PROTEOMICS Genome Mapping, Assembly, and Comparison ■ 243 Genome Mapping ■ 243 Genome Sequencing ■ 245 Genome Sequence Assembly ■ 246 Genome Annotation ■ 250 Comparative Genomics ■ 255 Summary ■ 259 Further Reading ■ 259 18 Functional Genomics ■ 261 Sequence-Based Approaches ■ 261 Microarray-Based Approaches ■ 267 Comparison of SAGE and DNA Microarrays ■ 278 Summary ■ 279 Further Reading ■ 280 19 Proteomics ■ 281 Technology of Protein Expression Analysis ■ 281 Posttranslational Modification ■ 287 Protein Sorting ■ 289 Protein–Protein Interactions ■ 291 Summary ■ 296 Further Reading ■ 296 APPENDIX Appendix 1. Practical Exercises ■ 301 Appendix 2. Glossary ■ 318 Index ■ 331 January 10, 2006 15:7 P1: JZP 0521840988pre CB1022/Xiong 0 521 84098 8 January 10, 2006 Preface With a large number of prokaryotic and eukaryotic genomes completely sequenced and more forthcoming, access to the genomic information and synthesizing it for the discovery of new knowledge have become central themes of modern biological research. Mining the genomic information requires the use of sophisticated computational tools. It therefore becomes imperative for the new generation of biologists to be familiar with many bioinformatics programs and databases to tackle the new challenges in the genomic era. To meet this goal, institutions in the United States and around the world are now offering graduate and undergraduate students bioinformatics-related courses to introduce them to relevant computational tools necessary for the genomic research. To support this important task, this text was written to provide comprehensive coverage on the state-of-the-art of bioinformatics in a clear and concise manner. The idea of writing a bioinformatics textbook originated from my experience of teaching bioinformatics at Texas A&M University. I needed a text that was comprehensive enough to cover all major aspects in the field, technical enough for a collegelevel course, and sufficiently up to date to include most current algorithms while at the same time being logical and easy to understand. The lack of such a comprehensive text at that time motivated me to write extensive lecture notes that attempted to alleviate the problem. The notes turned out to be very popular among the students and were in great demand from those who did not even take the class. To benefit a larger audience, I decided to assemble my lecture notes, as well as my experience and interpretation of bioinformatics, into a book. This book is aimed at graduate and undergraduate students in biology, or any practicing molecular biologist, who has no background in computer algorithms but wishes to understand the fundamental principles of bioinformatics and use this knowledge to tackle his or her own research problems. It covers major databases and software programs for genomic data analysis, with an emphasis on the theoretical basis and practical applications of these computational tools. By reading this book, the reader will become familiar with various computational possibilities for modern molecular biological research and also become aware of the strengths and weaknesses of each of the software tools. The reader is assumed to have a basic understanding of molecular biology and biochemistry. Therefore, many biological terms, such as nucleic acids, amino acids, genes, transcription, and translation, are used without further explanation. One exception is protein structure, for which a chapter about fundamental concepts is included so that ix 15:7 P1: JZP 0521840988pre x CB1022/Xiong 0 521 84098 8 January 10, 2006 PREFACE algorithms and rationales for protein structural bioinformatics can be better understood. Prior knowledge of advanced statistics, probability theories, and calculus is of course preferable but not essential. This book is organized into six sections: biological databases, sequence alignment, genes and promoter prediction, molecular phylogenetics, structural bioinformatics, and genomics and proteomics. There are nineteen chapters in total, each of which is relatively independent. When information from one chapter is needed for understanding another, cross-references are provided. Each chapter includes definitions and key concepts as well as solutions to related computational problems. Occasionally there are boxes that show worked examples for certain types of calculations. Since this book is primarily for molecular biologists, very few mathematical formulas are used. A small number of carefully chosen formulas are used where they are absolutely necessary to understand a particular concept. The background discussion of a computational problem is often followed by an introduction to related computer programs that are available online. A summary is also provided at the end of each chapter. Most of the programs described in this book are online tools that are freely available and do not require special expertise to use them. Most of them are rather straightforward to use in that the user only needs to supply sequences or structures as input, and the results are returned automatically. In many cases, knowing which programs are available for which purposes is sufficient, though occasionally skills of interpreting the results are needed. However, in a number of instances, knowing the names of the programs and their applications is only half the journey. The user also has to make special efforts to learn the intricacies of using the programs. These programs are considered to be on the other extreme of user-friendliness. However, it would be impractical for this book to try to be a computer manual for every available software program. That is not my goal in writing the book. Nonetheless, having realized the difficulties of beginners who are often unaware of or, more precisely, intimidated by the numerous software programs available, I have designed a number of practical Web exercises with detailed step-by-step procedures that aim to serve as examples of the correct use of a combined set of bioinformatics tools for solving a particular problem. The exercises were originally written for use on a UNIX workstation. However, they can be used, with slight modifications, on any operating systems with Internet access. In the course of preparing this book, I consulted numerous original articles and books related to certain topics of bioinformatics. I apologize for not being able to acknowledge all of these sources because of space limitations in such an introductory text. However, a small number of articles (mainly recent review articles) and books related to the topics of each chapter are listed as “Further Reading” for those who wish to seek more specialized information on the topics. Regarding the inclusion of computational programs, there are often a large number of programs available for a particular task. I apologize for any personal bias in the selection of the software programs in the book. 15:7 P1: JZP 0521840988pre CB1022/Xiong 0 521 84098 8 January 10, 2006 xi PREFACE One of the challenges in writing this text was to cover sufficient technical background of computational methods without extensive display of mathematical formulas. I strived to maintain a balance between explaining algorithms and not getting into too much mathematical detail, which may be intimidating for beginning students and nonexperts in computational biology. This sometimes proved to be a tough balance for me because I risk either sacrificing some of the original content or losing the reader. To alleviate this problem, I chose in many instances to use graphics instead of formulas to illustrate a concept and to aid understanding. I would like to thank the Department of Biology at Texas A&M University for the opportunity of letting me teach a bioinformatics class, which is what made this book possible. I thank all my friends and colleagues in the Department of Biology and the Department of Biochemistry for their friendship. Some of my colleagues were kind enough to let me participate in their research projects, which provided me with diverse research problems with which I could hone my bioinformatics analysis skills. I am especially grateful to Lisa Peres of the Molecular Simulation Laboratory at Texas A&M, who was instrumental in helping me set up and run the laboratory section of my bioinformatics course. I am also indebted to my former postdoctoral mentor, Carl Bauer of Indiana University, who gave me the wonderful opportunity to learn evolution and phylogenetics in great depth, which essentially launched my career in bioinformatics. Also importantly, I would like to thank Katrina Halliday, my editor at Cambridge University Press, for accepting the manuscript and providing numerous suggestions for polishing the early draft. It was a great pleasure working with her. Thanks also go to Cindy Fullerton and Marielle Poss for their diligent efforts in overseeing the copyediting of the book to ensure a quality final product. Jin Xiong 15:7 P1: JZP 0521840988pre CB1022/Xiong 0 521 84098 8 January 10, 2006 xii 15:7 P1: JZP 0521840988c01 CB1022/Xiong 0 521 84098 8 January 10, 2006 SECTION ONE Introduction and Biological Databases 1 9:48 P1: JZP 0521840988c01 CB1022/Xiong 0 521 84098 8 January 10, 2006 2 9:48 P1: JZP 0521840988c01 CB1022/Xiong 0 521 84098 8 January 10, 2006 CHAPTER ONE Introduction Quantitation and quantitative tools are indispensable in modern biology. Most biological research involves application of some type of mathematical, statistical, or computational tools to help synthesize recorded data and integrate various types of information in the process of answering a particular biological question. For example, enumeration and statistics are required for assessing everyday laboratory experiments, such as making serial dilutions of a solution or counting bacterial colonies, phage plaques, or trees and animals in the natural environment. A classic example in the history of genetics is by Gregor Mendel and Thomas Morgan, who, by simply counting genetic variations of plants and fruit flies, were able to discover the principles of genetic inheritance. More dedicated use of quantitative tools may involve using calculus to predict the growth rate of a human population or to establish a kinetic model for enzyme catalysis. For very sophisticated uses of quantitative tools, one may find application of the “game theory” to model animal behavior and evolution, or the use of millions of nonlinear partial differential equations to model cardiac blood flow. Whether the application is simple or complex, subtle or explicit, it is clear that mathematical and computational tools have become an integral part of modern-day biological research. However, none of these examples of quantitative tool use in biology could be considered to be part of bioinformatics, which is also quantitative in nature. To help the reader understand the difference between bioinformatics and other elements of quantitative biology, we provide a detailed explanation of what is bioinformatics in the following sections. Bioinformatics, which will be more clearly defined below, is the discipline of quantitative analysis of information relating to biological macromolecules with the aid of computers. The development of bioinformatics as a field is the result of advances in both molecular biology and computer science over the past 30–40 years. Although these developments are not described in detail here, understanding the history of this discipline is helpful in obtaining a broader insight into current bioinformatics research. A succinct chronological summary of the landmark events that have had major impacts on the development of bioinformatics is presented here to provide context. The earliest bioinformatics efforts can be traced back to the 1960s, although the word bioinformatics did not exist then. Probably, the first major bioinformatics project was undertaken by Margaret Dayhoff in 1965, who developed a first protein sequence database called Atlas of Protein Sequence and Structure. Subsequently, in the early 1970s, the Brookhaven National Laboratory established the Protein Data Bank for archiving three-dimensional protein structures. At its onset, the database stored less 3 9:48 P1: JZP 0521840988c01 4 CB1022/Xiong 0 521 84098 8 January 10, 2006 INTRODUCTION than a dozen protein structures, compared to more than 30,000 structures today. The first sequence alignment algorithm was developed by Needleman and Wunsch in 1970. This was a fundamental step in the development of the field of bioinformatics, which paved the way for the routine sequence comparisons and database searching practiced by modern biologists. The first protein structure prediction algorithm was developed by Chou and Fasman in 1974. Though it is rather rudimentary by today’s standard, it pioneered a series of developments in protein structure prediction. The 1980s saw the establishment of GenBank and the development of fast database searching algorithms such as FASTA by William Pearson and BLAST by Stephen Altschul and coworkers. The start of the human genome project in the late 1980s provided a major boost for the development of bioinformatics. The development and the increasingly widespread use of the Internet in the 1990s made instant access to, and exchange and dissemination of, biological data possible. These are only the major milestones in the establishment of this new field. The fundamental reason that bioinformatics gained prominence as a discipline was the advancement of genome studies that produced unprecedented amounts of biological data. The explosion of genomic sequence information generated a sudden demand for efficient computational tools to manage and analyze the data. The development of these computational tools depended on knowledge generated from a wide range of disciplines including mathematics, statistics, computer science, information technology, and molecular biology. The merger of these disciplines created an informationoriented field in biology, which is now known as bioinformatics. WHAT IS BIOINFORMATICS? Bioinformatics is an interdisciplinary research area at the interface between computer science and biological science. A variety of definitions exist in the literature and on the world wide web; some are more inclusive than others. Here, we adopt the definition proposed by Luscombe et al. in defining bioinformatics as a union of biology and informatics: bioinformatics involves the technology that uses computers for storage, retrieval, manipulation, and distribution of information related to biological macromolecules such as DNA, RNA, and proteins. The emphasis here is on the use of computers because most of the tasks in genomic data analysis are highly repetitive or mathematically complex. The use of computers is absolutely indispensable in mining genomes for information gathering and knowledge building. Bioinformatics differs from a related field known as computational biology. Bioinformatics is limited to sequence, structural, and functional analysis of genes and genomes and their corresponding products and is often considered computational molecular biology. However, computational biology encompasses all biological areas that involve computation. For example, mathematical modeling of ecosystems, population dynamics, application of the game theory in behavioral studies, and phylogenetic construction using fossil records all employ computational tools, but do not necessarily involve biological macromolecules. 9:48 P1: JZP 0521840988c01 CB1022/Xiong 0 521 84098 8 SCOPE Beside this distinction, it is worth noting that there are other views of how the two terms relate. For example, one version defines bioinformatics as the development and application of computational tools in managing all kinds of biological data, whereas computational biology is more confined to the theoretical development of algorithms used for bioinformatics. The confusion at present over definition may partly reflect the nature of this vibrant and quickly evolving new field. GOALS The ultimate goal of bioinformatics is to better understand a living cell and how it functions at the molecular level. By analyzing raw molecular sequence and structural data, bioinformatics research can generate new insights and provide a “global” perspective of the cell. The reason that the functions of a cell can be better understood by analyzing sequence data is ultimately because the flow of genetic information is dictated by the “central dogma” of biology in which DNA is transcribed to RNA, which is translated to proteins. Cellular functions are mainly performed by proteins whose capabilities are ultimately determined by their sequences. Therefore, solving functional problems using sequence and sometimes structural approaches has proved to be a fruitful endeavor. SCOPE Bioinformatics consists of two subfields: the development of computational tools and databases and the application of these tools and databases in generating biological knowledge to better understand living systems. These two subfields are complementary to each other. The tool development includes writing software for sequence, structural, and functional analysis, as well as the construction and curating of biological databases. These tools are used in three areas of genomic and molecular biological research: molecular sequence analysis, molecular structural analysis, and molecular functional analysis. The analyses of biological data often generate new problems and challenges that in turn spur the development of new and better computational tools. The areas of sequence analysis include sequence alignment, sequence database searching, motif and pattern discovery, gene and promoter finding, reconstruction of evolutionary relationships, and genome assembly and comparison. Structural analyses include protein and nucleic acid structure analysis, comparison, classification, and prediction. The functional analyses include gene expression profiling, protein– protein interaction prediction, protein subcellular localization prediction, metabolic pathway reconstruction, and simulation (Fig. 1.1). The three aspects of bioinformatics analysis are not isolated but often interact to produce integrated results (see Fig. 1.1). For example, protein structure prediction depends on sequence alignment data; clustering of gene expression profiles requires the use of phylogenetic tree construction methods derived in sequence analysis. Sequence-based promoter prediction is related to functional analysis of January 10, 2006 5 9:48 P1: JZP 0521840988c01 6 CB1022/Xiong 0 521 84098 8 January 10, 2006 INTRODUCTION Figure 1.1: Overview of various subfields of bioinformatics. Biocomputing tool development is at the foundation of all bioinformatics analysis. The applications of the tools fall into three areas: sequence analysis, structure analysis, and function analysis. There are intrinsic connections between different areas of analyses represented by bars between the boxes. coexpressed genes. Gene annotation involves a number of activities, which include distinction between coding and noncoding sequences, identification of translated protein sequences, and determination of the gene’s evolutionary relationship with other known genes; prediction of its cellular functions employs tools from all three groups of the analyses. APPLICATIONS Bioinformatics has not only become essential for basic genomic and molecular biology research, but is having a major impact on many areas of biotechnology and biomedical sciences. It has applications, for example, in knowledge-based drug design, forensic DNA analysis, and agricultural biotechnology. Computational studies of protein–ligand interactions provide a rational basis for the rapid identification of novel leads for synthetic drugs. Knowledge of the three-dimensional structures of proteins allows molecules to be designed that are capable of binding to the receptor site of a target protein with great affinity and specificity. This informatics-based approach 9:48 P1: JZP 0521840988c01 CB1022/Xiong 0 521 84098 8 LIMITATIONS significantly reduces the time and cost necessary to develop drugs with higher potency, fewer side effects, and less toxicity than using the traditional trial-and-error approach. In forensics, results from molecular phylogenetic analysis have been accepted as evidence in criminal courts. Some sophisticated Bayesian statistics and likelihood-based methods for analysis of DNA have been applied in the analysis of forensic identity. It is worth mentioning that genomics and bioinformtics are now poised to revolutionize our healthcare system by developing personalized and customized medicine. The high speed genomic sequencing coupled with sophisticated informatics technology will allow a doctor in a clinic to quickly sequence a patient’s genome and easily detect potential harmful mutations and to engage in early diagnosis and effective treatment of diseases. Bioinformatics tools are being used in agriculture as well. Plant genome databases and gene expression profile analyses have played an important role in the development of new crop varieties that have higher productivity and more resistance to disease. LIMITATIONS Having recognized the power of bioinformatics, it is also important to realize its limitations and avoid over-reliance on and over-expectation of bioinformatics output. In fact, bioinformatics has a number of inherent limitations. In many ways, the role of bioinformatics in genomics and molecular biology research can be likened to the role of intelligence gathering in battlefields. Intelligence is clearly very important in leading to victory in a battlefield. Fighting a battle without intelligence is inefficient and dangerous. Having superior information and correct intelligence helps to identify the enemy’s weaknesses and reveal the enemy’s strategy and intentions. The gathered information can then be used in directing the forces to engage the enemy and win the battle. However, completely relying on intelligence can also be dangerous if the intelligence is of limited accuracy. Overreliance on poor-quality intelligence can yield costly mistakes if not complete failures. It is no stretch in analogy that fighting diseases or other biological problems using bioinformatics is like fighting battles with intelligence. Bioinformatics and experimental biology are independent, but complementary, activities. Bioinformatics depends on experimental science to produce raw data for analysis. It, in turn, provides useful interpretation of experimental data and important leads for further experimental research. Bioinformatics predictions are not formal proofs of any concepts. They do not replace the traditional experimental research methods of actually testing hypotheses. In addition, the quality of bioinformatics predictions depends on the quality of data and the sophistication of the algorithms being used. Sequence data from high throughput analysis often contain errors. If the sequences are wrong or annotations incorrect, the results from the downstream analysis are misleading as well. That is why it is so important to maintain a realistic perspective of the role of bioinformatics. January 10, 2006 7 9:48 P1: JZP 0521840988c01 8 CB1022/Xiong 0 521 84098 8 January 10, 2006 INTRODUCTION Bioinformatics is by no means a mature field. Most algorithms lack the capability and sophistication to truly reflect reality. They often make incorrect predictions that make no sense when placed in a biological context. Errors in sequence alignment, for example, can affect the outcome of structural or phylogenetic analysis. The outcome of computation also depends on the computing power available. Many accurate but exhaustive algorithms cannot be used because of the slow rate of computation. Instead, less accurate but faster algorithms have to be used. This is a necessary trade-off between accuracy and computational feasibility. Therefore, it is important to keep in mind the potential for errors produced by bioinformatics programs. Caution should always be exercised when interpreting prediction results. It is a good practice to use multiple programs, if they are available, and perform multiple evaluations. A more accurate prediction can often be obtained if one draws a consensus by comparing results from different algorithms. NEW THEMES Despite the pitfalls, there is no doubt that bioinformatics is a field that holds great potential for revolutionizing biological research in the coming decades. Currently, the field is undergoing major expansion. In addition to providing more reliable and more rigorous computational tools for sequence, structural, and functional analysis, the major challenge for future bioinformatics development is to develop tools for elucidation of the functions and interactions of all gene products in a cell. This presents a tremendous challenge because it requires integration of disparate fields of biological knowledge and a variety of complex mathematical and statistical tools. To gain a deeper understanding of cellular functions, mathematical models are needed to simulate a wide variety of intracellular reactions and interactions at the whole cell level. This molecular simulation of all the cellular processes is termed systems biology. Achieving this goal will represent a major leap toward fully understanding a living system. That is why the system-level simulation and integration are considered the future of bioinformatics. Modeling such complex networks and making predictions about their behavior present tremendous challenges and opportunities for bioinformaticians. The ultimate goal of this endeavor is to transform biology from a qualitative science to a quantitative and predictive science. This is truly an exciting time for bioinformatics. FURTHER READING Attwood, T. K., and Miller, C. J. 2002. Progress in bioinformatics and the importance of being earnest. Biotechnol. Annu. Rev. 8:1–54. Golding, G. B. 2003. DNA and the revolution of molecular evolution, computational biology, and bioinformatics. Genome 46:930–5. Goodman, N. 2002. Biological data becomes computer literature: New advances in bioinformatics. Curr. Opin. Biotechnol. 13:68–71. 9:48 P1: JZP 0521840988c01 CB1022/Xiong 0 521 84098 8 FURTHER READING Hagen. J. B. 2000. The origin of bioinformatics. Nat. Rev. Genetics 1:231–6. Kanehisa, M., and Bork, P. 2003. Bioinformatics in the post-sequence era. Nat. Genet. 33 Suppl:305–10. Kim, J. H. 2002. Bioinformatics and genomic medicine. Genet. Med. 4 Suppl:62S–5S. Luscombe, N. M., Greenbaum, D., and Gerstein, M. 2001. What is bioinformatics? A proposed definition and overview of the field. Methods Inf. Med. 40:346–58. Ouzounis, C. A., and Valencia, A. 2003. Early bioinformatics: The birth of a discipline – A personal view. Bioinformatics 19:2176–90. January 10, 2006 9 9:48 P1: JZP 0521840988c02 CB1022/Xiong 0 521 84098 8 January 10, 2006 CHAPTER TWO Introduction to Biological Databases One of the hallmarks of modern genomic research is the generation of enormous amounts of raw sequence data. As the volume of genomic data grows, sophisticated computational methodologies are required to manage the data deluge. Thus, the very first challenge in the genomics era is to store and handle the staggering volume of information through the establishment and use of computer databases. The development of databases to handle the vast amount of molecular biological data is thus a fundamental task of bioinformatics. This chapter introduces some basic concepts related to databases, in particular, the types, designs, and architectures of biological databases. Emphasis is on retrieving data from the main biological databases such as GenBank. WHAT IS A DATABASE? A database is a computerized archive used to store and organize data in such a way that information can be retrieved easily via a variety of search criteria. Databases are composed of computer hardware and software for data management. The chief objective of the development of a database is to organize data in a set of structured records to enable easy retrieval of information. Each record, also called an entry, should contain a number of fields that hold the actual data items, for example, fields for names, phone numbers, addresses, dates. To retrieve a particular record from the database, a user can specify a particular piece of information, called value, to be found in a particular field and expect the computer to retrieve the whole data record. This process is called making a query. Although data retrieval is the main purpose of all databases, biological databases often have a higher level of requirement, known as knowledge discovery, which refers to the identification of connections between pieces of information that were not known when the information was first entered. For example, databases containing raw sequence information can perform extra computational tasks to identify sequence homology or conserved motifs. These features facilitate the discovery of new biological insights from raw data. TYPES OF DATABASES Originally, databases all used a flat file format, which is a long text file that contains many entries separated by a delimiter, a special character such as a vertical bar (|). Within each entry are a number of fields separated by tabs or commas. Except for the 10 14:42 P1: JZP 0521840988c02 CB1022/Xiong 0 521 84098 8 January 10, 2006 TYPES OF DATABASES raw values in each field, the entire text file does not contain any hidden instructions for computers to search for specific information or to create reports based on certain fields from each record. The text file can be considered a single table. Thus, to search a flat file for a particular piece of information, a computer has to read through the entire file, an obviously inefficient process. This is manageable for a small database, but as database size increases or data types become more complex, this database style can become very difficult for information retrieval. Indeed, searches through such files often cause crashes of the entire computer system because of the memory-intensive nature of the operation. To facilitate the access and retrieval of data, sophisticated computer software programs for organizing, searching, and accessing data have been developed. They are called database management systems. These systems contain not only raw data records but also operational instructions to help identify hidden connections among data records. The purpose of establishing a data structure is for easy execution of the searches and to combine different records to form final search reports. Depending on the types of data structures,these database management systems can be classified into two types: relational database management systems and object-oriented database management systems. Consequently, databases employing these management systems are known as relational databases or object-oriented databases, respectively. Relational Databases Instead of using a single table as in a flat file database, relational databases use a set of tables to organize data. Each table, also called a relation, is made up of columns and rows. Columns represent individual fields. Rows represent values in the fields of records. The columns in a table are indexed according to a common feature called an attribute, so they can be cross-referenced in other tables. To execute a query in a relational database, the system selects linked data items from different tables and combines the information into one report. Therefore, specific information can be found more quickly from a relational database than from a flat file database. Relational databases can be created using a special programming language called structured query language (SQL). The creation of this type of databases can take a great deal of planning during the design phase. After creation of the original database, a new data category can be easily added without requiring all existing tables to be modified. The subsequent database searching and data gathering for reports are relatively straightforward. Here is a simple example of student course information expressed in a flat file which contains records of five students from four different states, each taking a different course (Fig. 2.1). Each data record, separated by a vertical bar, contains four fields describing the name, state, course number and title. A relational database is also created to store the same information, in which the data are structured as a number of tables. Figure 2.1 shows how the relational database works. In each table, data that fit a particular criterion are grouped together. Different tables can be linked by common data categories, which facilitate finding of specific information. 11 14:42 P1: JZP 0521840988c02 12 CB1022/Xiong 0 521 84098 8 January 10, 2006 INTRODUCTION TO BIOLOGICAL DATABASES Figure 2.1: Example of constructing a relational database for five students’ course information originally expressed in a flat file. By creating three different tables linked by common fields, data can be easily accessed and reassembled. For example, if one is to ask the question, which courses are students from Texas taking? The database will first find the field for “State” in Table A and look up for Texas. This returns students 1 and 5. The student numbers are colisted in Table B, in which students 1 and 5 correspond to Biol 689 and Math 172, respectively. The course names listed by course numbers are found in Table C. By going to Table C, exact course names corresponding to the course numbers can be retrieved. A final report is then given showing that the Texans are taking the courses Bioinformatics and Calculus. However, executing the same query through the flat file requires the computer to read through the entire text file word by word and to store the information in a temporay memory space and later mark up the data records containing the word Texas. This is easily accomplishable for a small database. To perform queries in a large database using flat files obviously becomes an onerous task for the computer system. Object-Oriented Databases One of the problems with relational databases is that the tables used do not describe complex hierarchical relationships between data items. To overcome the problem, object-oriented databases have been developed that store data as objects. In an object-oriented programming language, an object can be considered as a unit that combines data and mathematical routines that act on the data. The database is structured such that the objects are linked by a set of pointers defining predetermined relationships between the objects. Searching the database involves navigating through the objects with the aid of the pointers linking different objects. Programming languages like C++ are used to create object-oriented databases. The object-oriented database system is more flexible; data can be structured based on hierarchical relationships. By doing so, programming tasks can be simplified for data that are known to have complex relationships, such as multimedia data. However, 14:42 P1: JZP 0521840988c02 CB1022/Xiong 0 521 84098 8 January 10, 2006 BIOLOGICAL DATABASES Figure 2.2: Example of construction and query of an object-oriented database using the same student information as shown in Figure 2.1. Three objects are constructed and are linked by pointers shown as arrows. Finding specific information relies on navigating through the objects by way of pointers. For simplicity, some of the pointers are omitted. this type of database system lacks the rigorous mathematical foundation of the relational databases. There is also a risk that some of the relationships between objects may be misrepresented. Some current databases have therefore incorporated features of both types of database programming, creating the object–relational database management system. The above students’ course information (Fig. 2.1) can be used to construct an object-oriented database. Three different objects can be designed: student object, course object, and state object. Their interrelations are indicated by lines with arrows (Fig. 2.2). To answer the same question – which courses are students from Texas taking – one simply needs to start from Texas in the state object, which has pointers that lead to students 1 and 5 in the student object. Further pointers in the student object point to the course each of the two students is taking. Therefore, a simple navigation through the linked objects provides a final report. BIOLOGICAL DATABASES Current biological databases use all three types of database structures: flat files, relational, and object oriented. Despite the obvious drawbacks of using flat files in database management, many biological databases still use this format. The justification for this is that this system involves minimum amount of database design and the search output can be easily understood by working biologists. 13 14:42 P1: JZP 0521840988c02 14 CB1022/Xiong 0 521 84098 8 January 10, 2006 INTRODUCTION TO BIOLOGICAL DATABASES Based on their contents, biological databases can be roughly divided into three categories: primary databases, secondary databases, and specialized databases. Primary databases contain original biological data. They are archives of raw sequence or structural data submitted by the scientific community. GenBank and Protein Data Bank (PDB) are examples of primary databases. Secondary databases contain computationally processed or manually curated information, based on original information from primary databases. Translated protein sequence databases containing functional annotation belong to this category. Examples are SWISS-Prot and Protein Information Resources (PIR) (successor of Margaret Dayhoff’s Atlas of Protein Sequence and Structure [see Chapter 1]). Specialized databases are those that cater to a particular research interest. For example, Flybase, HIV sequence database, and Ribosomal Database Project are databases that specialize in a particular organism or a particular type of data. A list of some frequently used databases is provided in Table 2.1. Primary Databases There are three major public sequence databases that store raw nucleic acid sequence data produced and submitted by researchers worldwide: GenBank, the European Molecular Biology Laboratory (EMBL) database and the DNA Data Bank of Japan (DDBJ), which are all freely available on the Internet. Most of the data in the databases are contributed directly by authors with a minimal level of annotation. A small number of sequences, especially those published in the 1980s, were entered manually from published literature by database management staff. Presently, sequence submission to either GenBank, EMBL, or DDBJ is a precondition for publication in most scientific journals to ensure the fundamental molecular data to be made freely available. These three public databases closely collaborate and exchange new data daily. They together constitute the International Nucleotide Sequence Database Collaboration. This means that by connecting to any one of the three databases, one should have access to the same nucleotide sequence data. Although the three databases all contain the same sets of raw data, each of the individual databases has a slightly different kind of format to represent the data. Fortunately, for the three-dimensional structures of biological macromolecules, there is only one centralized database, the PDB. This database archives atomic coordinates of macromolecules (both proteins and nucleic acids) determined by x-ray crystallography and NMR. It uses a flat file format to represent protein name, authors, experimental details, secondary structure, cofactors, and atomic coordinates. The web interface of PDB also provides viewing tools for simple image manipulation. More details of this database and its format are provided in Chapter 12. Secondary Databases Sequence annotation information in the primary database is often minimal. To turn the raw sequence information into more sophisticated biological knowledge, much postprocessing of the sequence information is needed. This begs the need for 14:42 P1: JZP 0521840988c02 CB1022/Xiong 0 521 84098 8 January 10, 2006 15 BIOLOGICAL DATABASES TABLE 2.1. Major Biological Databases Available Via the World Wide Web Databases and Retrieval Systems AceDB DDBJ EMBL Entrez ExPASY FlyBase FSSP GenBank HIV databases Microarray gene expression database OMIM PIR PubMed Ribosomal database project SRS SWISS-Prot TAIR Brief Summary of Content URL Genome database for Caenorhabditis elegans Primary nucleotide sequence database in Japan Primary nucleotide sequence database in Europe NCBI portal for a variety of biological databases Proteomics database A database of the Drosophila genome Protein secondary structures Primary nucleotide sequence database in NCBI HIV sequence data and related immunologic information DNA microarray data and analysis tools Genetic information of human diseases Annotated protein sequences Biomedical literature information Ribosomal RNA sequences and phylogenetic trees derived from the sequences General sequence retrieval system Curated protein sequence database Arabidopsis information database secondary databases, which contain computationally processed sequence information derived from the primary databases. The amount of computational processing work varies greatly among the secondary databases; some are simple archives of translated sequence data from identified open reading frames in DNA, whereas others provide additional annotation and information related to higher levels of information regarding structure and functions. A prominent example of secondary databases is SWISS-PROT, which provides detailed sequence annotation that includes structure, function, and protein family assignment. The sequence data are mainly derived from TrEMBL, a database of 14:42 P1: JZP 0521840988c02 16 CB1022/Xiong 0 521 84098 8 January 10, 2006 INTRODUCTION TO BIOLOGICAL DATABASES translated nucleic acid sequences stored in the EMBL database. The annotation of each entry is carefully curated by human experts and thus is of good quality. The protein annotation includes function, domain structure, catalytic sites, cofactor binding, posttranslational modification, metabolic pathway information, disease association, and similarity with other sequences. Much of this information is o